Running AI in Production? With Great Power comes Great Responsibility
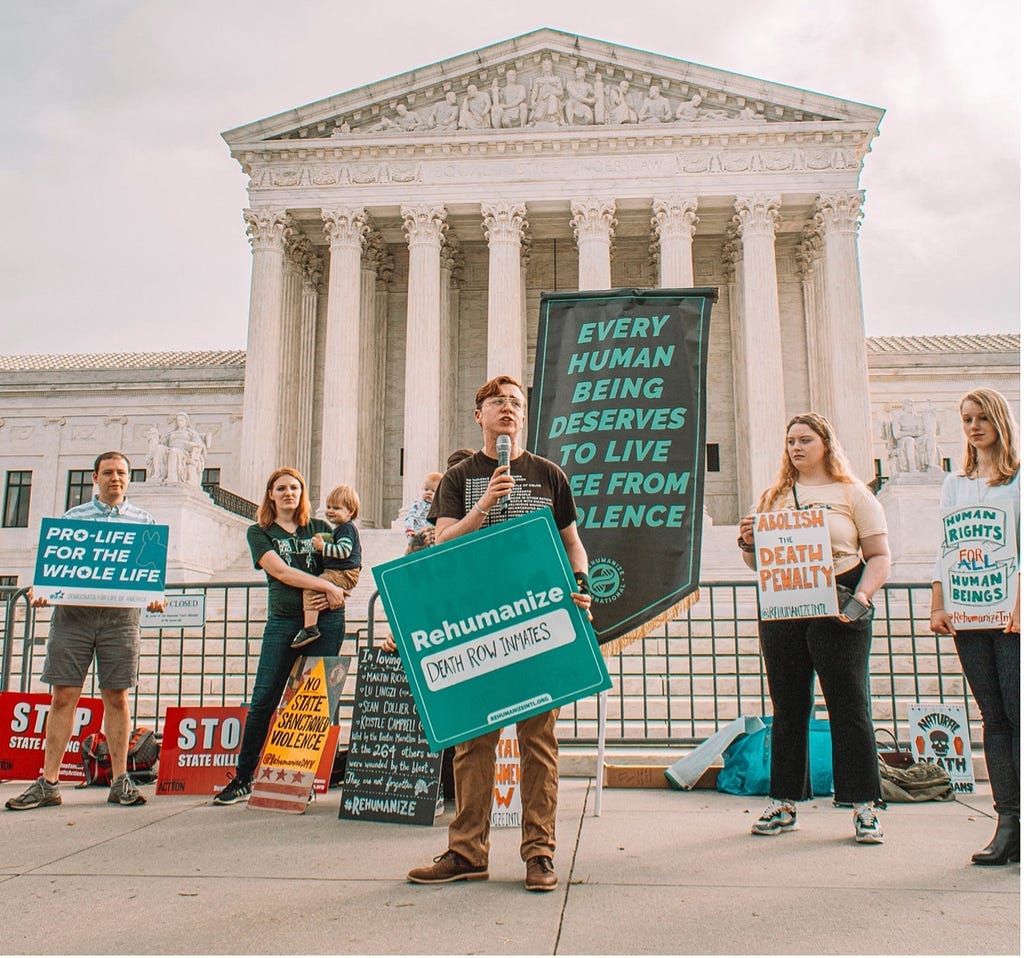
Nice! You finally did it! You’re one pipeline deployment away from putting your first AI model into production.
You conquered all the mighty battles. Not only you managed to get your data quality up to par, hired a small army of brilliant data scientists, and convinced the IT crowd to give you access to a few pieces of scalable infrastructure to crunch the numbers and make that high profile AI project actually happen.
Before uncorking the champagne bottles, that have been chilling in the fridge of that board room for ages. Please hold your horses, and don’t forget that champagne is often released under high pressure.
When you finally open those bottles, it’s better to have that white Napkin ready to make sure you don’t smutch those expensive leather chairs!
Your AI initiative is not ready for production, as long as you didn’t carefully define and implement the core principles to ensure that you are using A.I. in an ethical, human-first and sustainable way.
Here are 9 principles, to get you started:
Principle 1: AI Register
Category: Transparency & Openness
All AI-based algorithms are documented in the company’s AI register. The register is publicly accessible. For each algorithm, the following aspects need to be documented:
Key data sources that are utilised in the development and use of the system, context, and utilisation methods.
The operational logic of the data processing and reasoning performed by the system and the models used.
Explanation of how the algorithm promotes and realises equality principles to avoid discrimination.
The way human oversight is applied during the use of the service.
Risk assessment and mitigation practices.
Each aspect needs to refer to its related principle within the AI Ethics policy, which is published on the companies’ public website.
Principle 2: Privacy-by-Design
Category: Privacy Protection
Each algorithm must adhere to the privacy-by-design principles.
All algorithms need to be evaluated by using a Privacy Impact Assessment Artifact.
The personal data and the context in which it is used needs to be clearly documented and follow the GDPR guidelines.
Principle 3: AI Ethics Board
Category: Governance, Accountability, Bias and Regulatory Compliance
An AI Ethics board is appointed responsible for defining and governing the Ethical AI principles and their practical application. The board needs to comprise members whose age-, gender- and ethnic diversification reflects the distribution among the companies’ employees and customers as much as possible.
The board is charged with:
Conducting regular risk assessments, approving algorithms before they are used in a production context, including potential biases in their data sets and the mechanisms put in place to avoid decision skew due to outdated models.
Assuring that the organisations’ senior management knows and accepts eventual risks associated with the algorithm and acknowledges full accountability for any issue that might arise from deploying the algorithm within their organisation.
Continually evaluating new regulations and insights related to AI ethics within society. Updating the AI Ethics policy whenever necessary.
Assessing the ethical implications of AI algorithms from a holistic point of view. This means the impact of all algorithms as a whole and their interactions within the organisation’s ecosystem.
Principle 4: Evaluation of Human Autonomy & Digital Wellbeing
Category: Human Autonomy
All algorithms need to be evaluated through the 6 Spheres of Technology Experience based on the METUX method.
The analysis, tradeoffs, and decisions based on the research need to be documented and signed off by the AI Ethics Board.
Principle 5: Human Control and Right for Rectification
Category: Human Oversight and Replication
Each algorithm involved in human-oriented decision-making processes should allow for the right of rectification. It should provide precise mechanisms for the persona impacted by the decision-making process to request a re-evaluation of the decision by a human agent and is entitled to explain the main factors involved in the generation of such decision.
Each persona impacted by such a decision-making process has the right to submit new or additional data when relevant to decision making.
The right for rectification is to be interpreted in the context of the principles of proportionality.
Principle 6: Obligation to report non-ethical behaviour
Category: Protection of whistleblowers
Each employee is obliged to report non-ethical behaviour concerning the development of or experimentation with AI algorithms.
Such a report can be made to the AI Ethics board, or when a conflict of interest is suspected, it can be submitted to the appropriate government institution.
An employee reporting such a violation is protected against being fired for one year after the investigation’s closure.
Principle 7: AI Ethics awareness
Category: Awareness
All employees directly or indirectly involved in defining the algorithmic models, defining and selecting the algorithmic data sets, or applying AI algorithms should follow bi-yearly AI Ethics awareness training.
Principle 8: Safety and Cybersecurity
Category: Security
The Technical and Organisational measures to protect the confidentiality, integrity, and availability of the source data sets used by the algorithm, the algorithm itself and any insights generated by the algorithm should be assessed using the CARE standard of cybersecurity.
The cybersecurity measures and security risk management processes instituted within the organisation are also applicable to AI algorithms. Either created internally or sourced from third parties.
AI-specific cybersecurity KPI’s need to be defined and are integrated within the organisations’ Information Security Management System.
Principle 9: Inclusion and Diversity
Category: Solidarity, Inclusion and Social Cohesion
Any algorithm is to be tested and verified to take into account minority groups properly.
All bias, under-or overrepresentation related to age, race, gender, politic, or social classification is to be removed from the algorithm’s decision logic.
Algorithms that do not meet these guidelines are not allowed to be used in a production context.
Embed your Principles in the Fabric of your Organisation
Putting these key Principles on paper is the easy part. Ensuring that they don’t become dead lettered, is where things become a tidbit more difficult.
Stand by your principles at all cost, and make sure to embed them within the fabric of your organisation.
References
Ainowinstitute.org. 2021. AI Now Report 2019. [online] Available at: <https://ainowinstitute.org/AI_Now_2019_Report.pdf> [Accessed 11 January 2021].
Calvo, R., Peters, D., Vold, K. and Ryan, R., 2021. Supporting Human Autonomy In AI Systems: A Framework For Ethical Enquiry. [online] Available at: <https://link.springer.com/chapter/10.1007%2F978-3-030-50585-1_2> [Accessed 11 January 2021].
Hagendorff, T., 2021. The Ethics Of AI Ethics: An Evaluation Of Guidelines. [online] Available at: <https://doi.org/10.1007/s11023-020-09517-8> [Accessed 11 January 2021].
Jobin, A., Ienca, M. and Vayena, E., 2021. The Global Landscape Of AI Ethics Guidelines. [online] Available at: <https://www.nature.com/articles/s42256-019-0088-2> [Accessed 11 January 2021].
MIAI. 2021. Amsterdam And Helsinki Launch Algorithm And AI Register — MIAI. [online] Available at: <https://ai-regulation.com/amsterdam-and-helsinki-launch-algorithm-and-ai-register/> [Accessed 11 January 2021].
Running AI in Production? With Great Power comes Great Responsibility was originally published in Data Arena on Medium, where people are continuing the conversation by highlighting and responding to this story.